FDL Europe 2022 - Live Twin: Hydrological Models
Can data-driven ML models, informed by real-time satellite data build an end-to-end flood prediction system?
Published 12 JAN 2023
Frontier Development Lab (FDL) is a public-private partnership with ESA in Europe and NASA in the USA. FDL works with commercial partners to apply AI technologies to space science, to push the frontiers of research and develop new tools to help solve some of the biggest challenges that humanity faces. These range from the effects of climate change to predicting space weather, from improving disaster response, to identifying meteorites that could hold the key to the history of our universe.
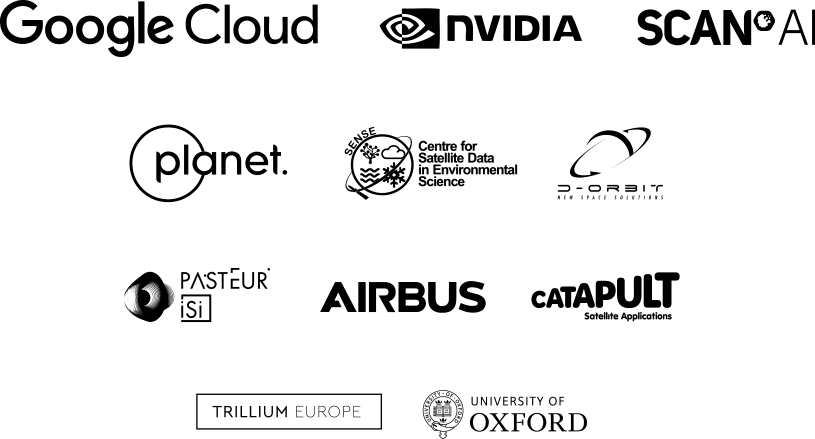
FDL Europe 2022 was a research sprint hosted by the University of Oxford that took place over a period of eight weeks in order to promote rapid learning and research outcomes in a collaborative atmosphere, pairing machine learning expertise with AI technologies and space science. The interdisciplinary teams address tightly defined problems and the format encourages rapid iteration and prototyping to create meaningful outputs to the space program and humanity.
Live Twin - Hydrological Models
Project Background
Floods can have a devastating effect on human lives, nature, and economies - between 1995 and 2015 over 2.6 billion people were affected by floods, comprising 56% of total people affected by weather related disasters. As flood phenomena become increasingly frequent and severe, better preparedness and mitigation strategies become necessary. According to the United Nations, reliable 72-hours-ahead predictions of river floods are vital, as they allow emergency agencies sufficient time to prepare, plan their mitigation strategies and deploy response teams on site. Such river flood prediction models already exist and perform relatively well in most high-income countries. However, these models are lacking in low-income countries due to limited data availability, where there is often the greatest flooding risk.
In recent years there have been initiatives to establish CAMELS (Catchment Attributes and Meteorology for Large-sample Studies) datasets at national levels and the UK, USA Australia, Chile and Brazil have these CAMELS datasets readily available - however they are not standardised and do not share the same attributes. More recently the Caravans (named after a series of camels) dataset has been published in an attempt to bring all other hydrological data into a single standard format with shared features and global coverage. This Caravans dataset was the starting point for the FDL team to set about developing the first end-to-end global river flood prediction framework, and its coverage is illustrated in the diagrams below.
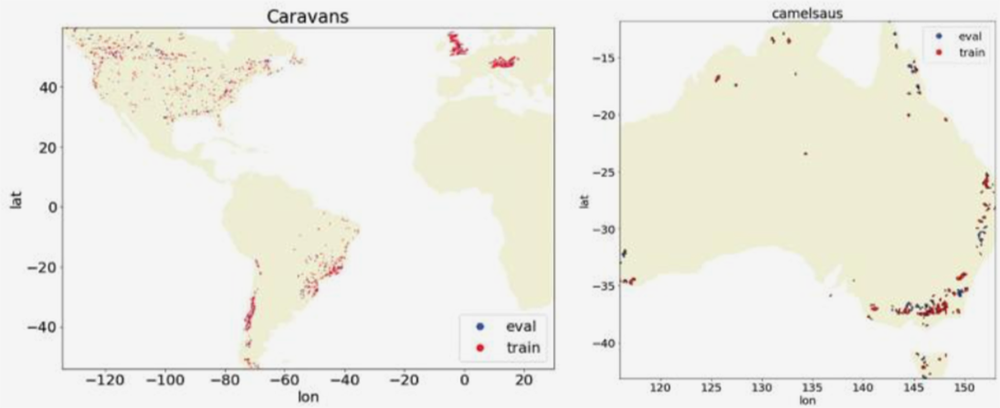
For each of the highlighted river basins on the maps, data includes a time series of gauge measured streamflow, 40 ERA5 (the ECMWF or European Centre for Medium Range Weather Forecasts Re-Analysis in its 5th generation) dynamic variables and corresponding climatic indices; and a collection of static attributes from HydroATLAS labs.
Project Approach
As the Caravans dataset doesn’t contain information for Africa or Asia, where a great number of flood-susceptible countries are located, the FDL team sought to expand the Caravans scope with an additional 195 random locations across these continents, as shown in the below diagram. As accurate streamflow measurements were not available for these locations, discharge figures, in m3/s, from the Global Runoff Data Centre (GRDC) were used and then converted to streamflow measurements, in mm/day, by analysing the available basin shape files from ERA5.
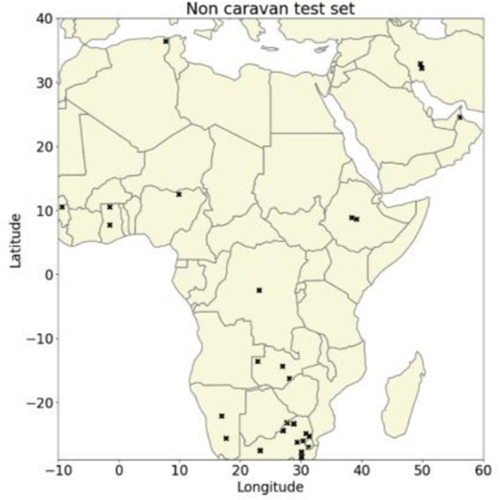
Using these newly calculated datasets, a pipeline was developed that covered all the necessary steps to obtain ML-ready data from the raw hydrological dataset - linear regression, random forest regression and deep Markov Chain neural networks. This preprocessing pipeline was designed to be highly flexible allowing for chunking and splitting of the data. The data loader is also flexible so models can be trained with different partitions and learning settings. Finally, a novel neural network Long Short Term Memory (LSTM) architecture was then designed that focused on a separate treatment of static and dynamic inputs. The dynamic variables include time series information, such as meteorological forcing and hydrological signatures, while the static variables include catchment attributes and climatic indices. This two path Long Short Term Memory (2P-LSTM) network is shown below.
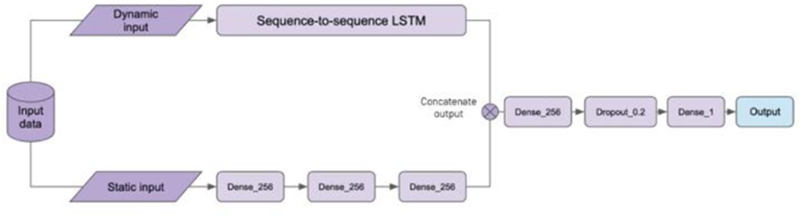
Machine learning benchmark models were then generated that targeted two important goals - firstly, three days ahead streamflow prediction in known basins and climate zones and secondly, spatial generalisability so the models can be applied to unseen basins and unseen climate zones. The resulting streamflow evaluations and predictions were pulled together to form floodcast AI - the first benchmark pipeline for global river flood predictions.
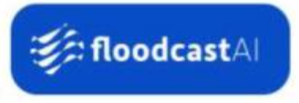
0 Days ahead
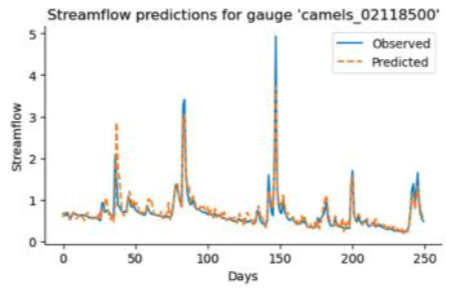
1 Days ahead
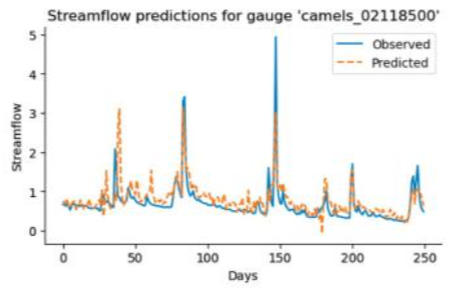
2 Days ahead
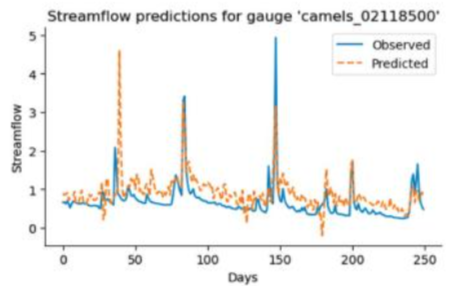
3 Days ahead
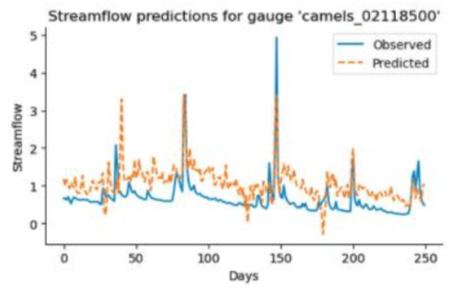
Project Results
The Hydrological models FDL team was able to predict with good accuracy the flood risk one day ahead of time. The models also demonstrated accurate high peak flow locations although the magnitude of these peaks was not so well defined. With unseen basins and climate zones the models also performed well, supporting the generalisability claim and the need to train models with globally available data. Further work will focus on whether training data from satellites could be replaced with forecasted data for inference, as this may improve accuracy and length of prediction window. You can learn more about this case study by reading the FDL 2022 Research Booklet, where a poster and full Technical Memorandum can also be downloaded.
The Scan Partnership
NVIDIA is a key supporter of the Frontier Development Lab and the FDL Europe 2022 event, and Scan AI was asked to act as a technology partner of NVIDIA to provide access to multiple DGX appliances in order to facilitate much of the machine learning and deep learning development and training required. The FDL Aerosols team used Google Cloud Platform (GCP) instances to prototype their models, prior to trained models then being deployed on a NVIDIA DGX platform in order to accelerate time to results.
“This is the third consecutive year we’ve working with NVIDIA to support the European FDL event and we are already committed to be part of the infrastructure for next years’ research sprint. It is a huge privilege to be associated with such ground-breaking research in light of the challenges we all face when it comes to climate change.”
Dan Parkinson, Director of Collaboration - Scan
Related content
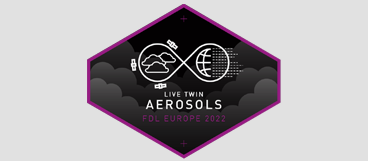
FDL Europe 2022 - Aerosols
Can ML techniques understand how aerosols from extreme fires in our warming world affect our weather and climate?
Read more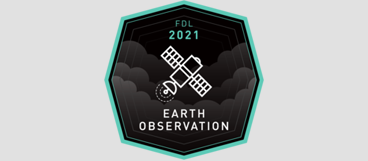
FDL Europe 2021 - World Food Embeddings
Using AI and satellite imagery to track the world’s food supply.
Read more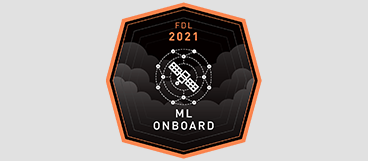
FDL Europe 2021 - ML Onboard
Using AI and satellite imagery to identify extreme weather ‘hot spots’.
Read more