FDL Europe 2021 - Extreme Event Hot Spots using ML Onboard
Can onboard AI technology be deployed on satellites to improve observations on extreme weather events?
Published 12 SEP 2022
Frontier Development Lab (FDL) is a public-private partnership with ESA in Europe and NASA in the USA. FDL works with commercial partners to apply AI technologies to space science, to push the frontiers of research and develop new tools to help solve some of the biggest challenges that humanity faces. These range from the affects of climate change to predicting space weather, from improving disaster response, to identifying meteorites that could hold the key to the history of our universe.
FDL Europe 2021 was a research sprint hosted by the University of Oxford, that takes place over a period of eight weeks in order to promote rapid learning and research outcomes in a collaborative atmosphere. This case study has been enabled by FDL alongside Phi-Lab (ESRIN) and ESA Mission Operations (ESOC), Trillium Technologies and the University of Oxford; the project has been also supported by Google Cloud, D-Orbit and Planet.
Project Background
Satellite observations of the Earth’s surface provide a vital data source for diverse environmental applications, including disaster management, landcover change detection and ecological monitoring. Currently, sensors on satellites collect and downlink all data points for further processing on the ground; yet, limitations in downlink capacity and speed result in delayed data availability and inefficient use of ground stations. These limitations affect time-sensitive applications such as disaster management where data is required with as low latency as possible to inform decision making in real time. This problem is set to worsen as the sensing resolution and number of satellites in orbit increase together with further restrictions on radio-frequency spectrum sharing licensing.
A solution is to apply processing onboard to identify the most useful data for a particular scenario, and prioritise this for rapid downlink. There has been considerable recent interest in using machine learning onboard for this processing. Existing work has focused on the deployment of supervised classifiers for applications such as identifying clouds or floods. Supervised learning has a significant drawback: only events of a particular type determined at training time will be flagged and prioritised for downlink, with no generalisation to new event types, imager specifications and lighting or local features.
Project Approach
In this work the ML Onboard project team proposed a new fully unsupervised novelty-detection model suitable for deployment on remote sensing platforms using a Variational AutoEncoder (VAE) to generate a latent representation of incoming sensor data over a particular region. A novelty score is assigned to this data using the distance in the latent space between representations from consecutive passes. This offers a substantial advantage over existing supervised methods as any change between passes can be detected onboard at inference time, regardless of the availability of training data.
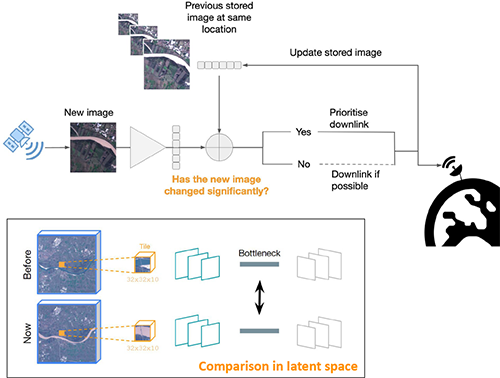
From these measurements, the team developed the RaVÆn library for unsupervised change detection for use onboard satellites. As shown in the table below, the team found that the VAE models (blue background) outperform a random baseline for all classes of event - landslides, hurricanes, wildfires and floods; and a custom baseline constructed by taking the cosine distance in pixel space for all classes except landslides. The scores in bold represent the best outcome for each class of event depending on the dimensions of the latent space.
Percentage of events identified | Landslides | Hurricanes | Wildfires | Floods |
---|---|---|---|---|
Random Baseline | 33.62% | 43.69% | 65.70% | 30.06% |
Custom baseline: Cosine distance | 60.19% | 59.58% | 87.50% | 38.18% |
VAE100 | 59.02% | 74.96% | 90.83% | 43.11% |
VAE1024 | 58.91% | 74.85% | 89.91% | 46.04% |
Project Results
Traditionally, data analysis is performed on the ground after all data is transferred – downlinked – to a ground station. Constraint on the downlink capabilities therefore affects any downstream application. In contrast, RaVÆn pre-processes the sampled data directly on the satellite and flags changed areas to prioritise for downlink, shortening the response time. The team verified the efficacy of the system on a dataset composed of time series of catastrophic events, demonstrating that RaVÆn outperforms pixel-wise baselines.
The results from the VAE model allows the creation of change-score maps, that show a qualitative comparison between, for example, before a river that floods or a wildfire occurs and afterwards showing a change in colour in the after image. In these examples - the ML Onboard method – the cosine embedding produces a change map that is crisper than the cosine baseline; for instance, the small flooded canal can be seen in the cosine embedding image but not in the baseline.
Flooding Example

Wildfire Example
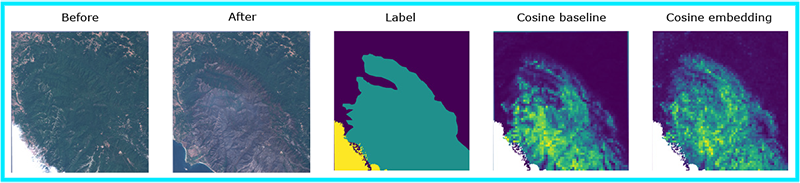
Conclusion
In conclusion, the ML Onboard team introduce a new method - RaVÆn - for unsupervised change detection in remote sensing data using a VAE. The team’s method was evaluated on a new dataset of remote sensing images of disasters. The proposed model outperforms a classical computer vision baseline in all of the tested disaster classes. This demonstrates that RaVÆn is a robust change detection method and suitable for application in improving data acquisition for disaster response.
You can learn more about this case study by reading the FDL 2021 Research Booklet , where a full Technical Memorandum can also be downloaded.
The Scan Partnership
Scan and NVIDIA are keen supporters of the Frontier Development Lab and the FDL Europe 2021 event provided access to multiple NVIDIA DGX AI systemss in order to facilitate much of the machine learning and deep learning development and training required. The ML Onboard project team used Google Cloud Platform (GCP) instances to prototype their models, prior to trained models then being deployed on a NVIDIA DGX platform in order to accelerate time to results.
‘Being able to support NVIDIA and the FDL research event is a huge honour as the project focus tackles challenges that affect the planet now and how we respond to them’
Dan Parkinson, Director of Collaboration - Scan Computers
Related content
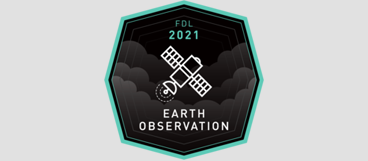
FDL Europe 2021 - World Food Embeddings
Using AI and satellite imagery to track the world’s food supply.
Read more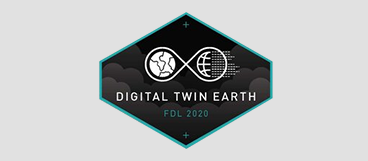
FDL Europe 2020 - Digital Twin Earth
Machine learning to make rain forecasting more accurate.
Read more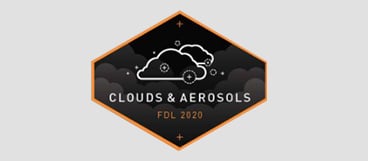
FDL Europe 2020 - Clouds & Aerosols
Aerosol effects on mesoscale cloud structures in marine boundary layer clouds
Read more