The AI Centre for Value-Based Healthcare (AI Centre) is a consortium of academic, NHS and industry partners led by King's College London (KCL) and based at St Thomas' Hospital. The AI Centre's partner organisations include University College London (UCL), Imperial College London, Queen Mary's University London and the Health Innovation Network, supported by numerous industry partners including Scan and NVIDIA.
The diverse research teams within the AI Centre develop and train sophisticated AI algorithms from a vast wealth of NHS medical images and patient pathway data to create new healthcare tools powered by federated learning, to provide faster diagnosis, personalised therapies and effective screening across a range of conditions and procedures. AI algorithms perform better when they have more data to train on, but it must be remembered healthcare patient data is highly confidential and sensitive.

Proof of Concept
In July 2019, Scan brought together NVIDIA, Mellanox and NetApp at Kings College London to design a GPU-accelerated compute cluster to develop AI-powered medical imaging at the point of care. In contrast to traditional medical testing, which involves sending scans for further analysis by specialists, point of care testing allows the results gained from X-rays, CT scans or MRI to be delivered and analysed immediately, in real-time with the patient-clinician interaction. The proof of concept (POC) was two-fold; firstly, to determine the shortest time frames in which images could be analysed and presented to clinicians; and secondly to establish a minimal viable product (MVP) for future roll-out to other London-based NHS Trusts.
The finalised POC cluster employed an NVIDIA DGX-2 AI supercomputer with InfiniBand switches connecting to a NetApp AFF A800 all-flash storage array. Delivering two petaFLOPs of GPU compute power, the cluster enabled the team to upload large and varied image datasets and train an AI analysis and diagnostic model in timeframes of minutes rather than days. You can learn more about the system design and approach in the video below.
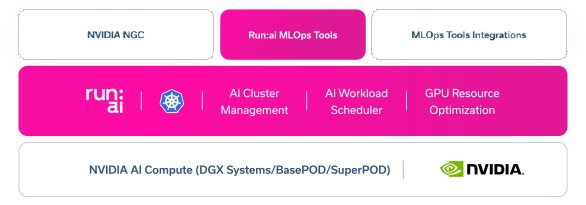
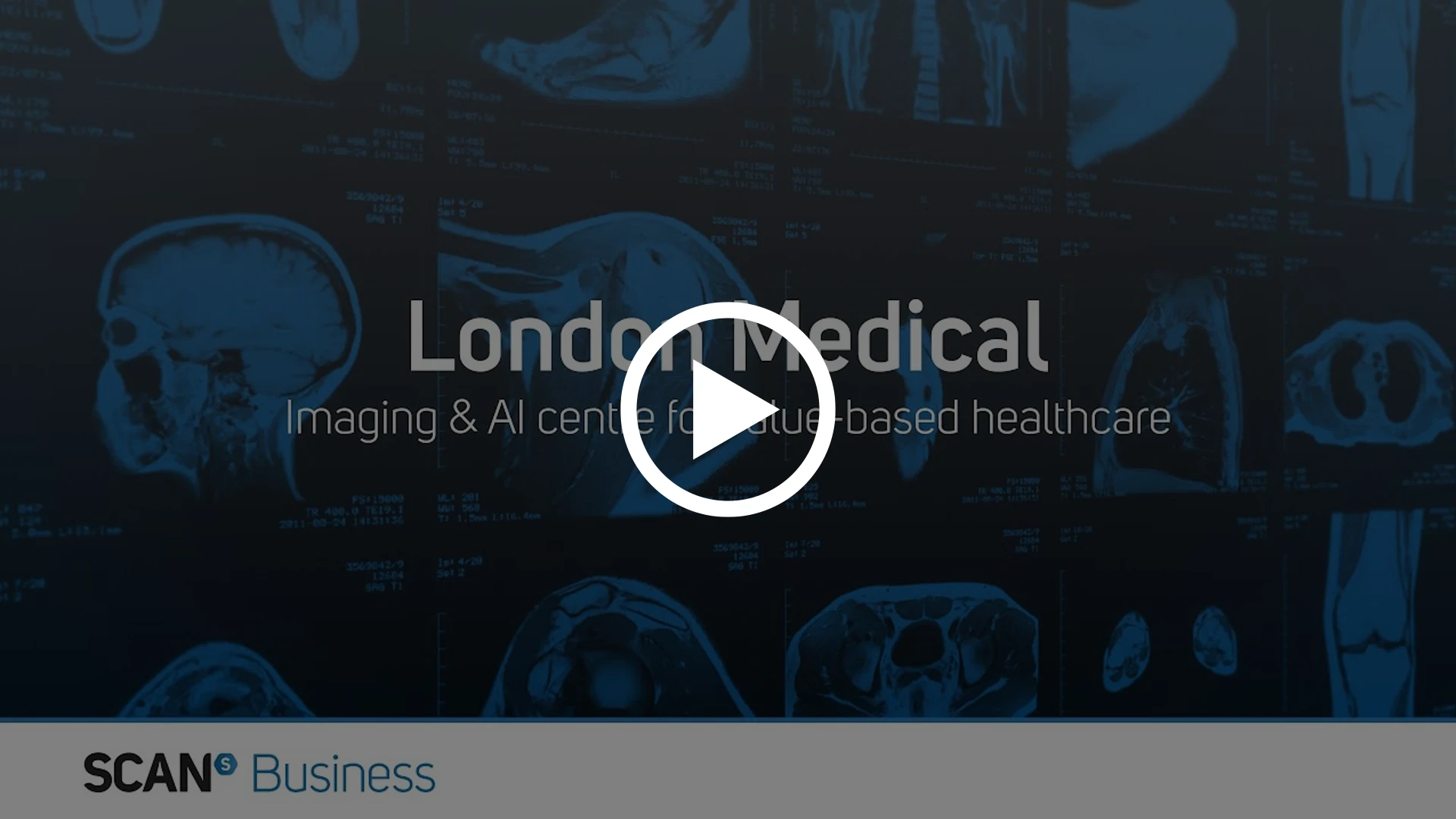
Following the validation of proof of concept system design it was decided to add a Run:ai software layer onto the cluster to further increase GPU utilisation. Run:ai enables flexible resource management and consumption so that users can easily access GPU fractions, multiple GPUs or clusters of GPUs for workloads of every size and stage of the AI lifecycle. This ensured that all available compute resources can be utilised and GPUs were never under-utilised, and gave the KCL AI Centre team control and real-time visibility over resource provisioning and queuing.
The Artificial Intelligence Deployment Engine
Following the successful proof of concept, the AI Centre received a £16m grant in August 2020, from the Department of Health and Social Care - Office for Life Sciences, to develop the initial concept into a wider multi-NHS Trust rollout. The proof of concept success resulted in a reference cluster design of an NVIDIA DGX A100, an 3XS EGX A30 and a 210TB NetApp AFF A800 storage array. This hardware in combination with the MONAI framework, supported the development of the Artificial Intelligence Development Engine (AIDE) firmware, installed on the first cluster at King's College Hospital NHS Foundation Trust in October 2021.
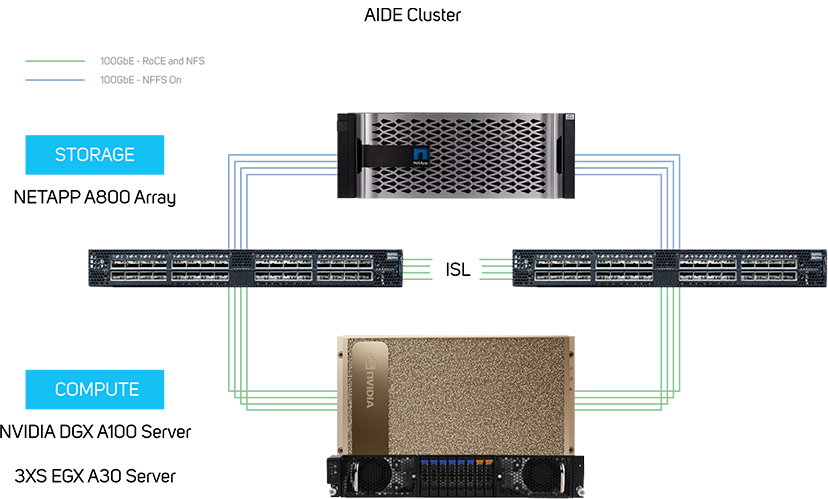
AIDE connects to the entire patient record and provides a harmonised hardware and software layer that facilitates the deployment and use of any AI product. Once clinical data has been analysed, the results will be sent to the electronic patient record to support clinical decision making - as the platform can receive a live stream of clinical data, it will allow clinicians to access near real-time AI analysis within seconds. The King's College Hospital Trust elected to initially deploy a stroke AI tool to support NHS clinicians and improve direct patient care, however the AI Centre developed further algorithms to support pathways such as dementia, heart failure and several cancers, prior to rollout of AIDE at other Trusts.
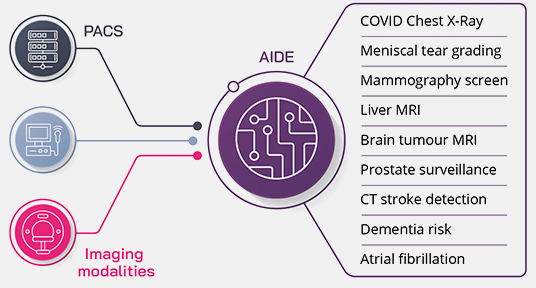
In October 2021 AIDE clusters were also installed at University College London NHS Foundation Trust and at Guy's and St Thomas' NHS Foundation Trust, followed by Imperial College Healthcare NHS Trust in January 2022, and University Hospital Sussex NHS Foundation Trust in February 2022.
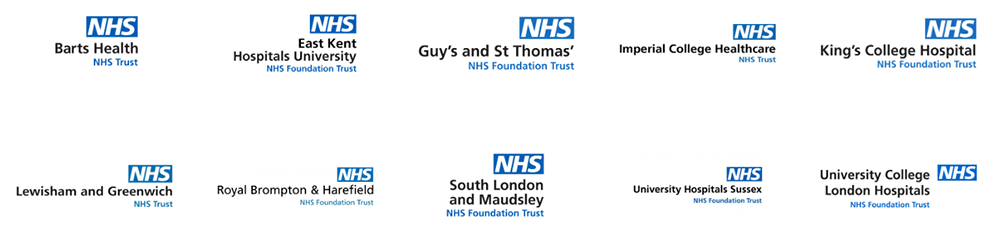
Next Steps
Following rollout of AIDE clusters to the five NHS Trusts, collectively serving over 10 million patients, the AI Centre was working to accelerate other AI models by combining the patient data from each of the separate AIDE clusters, without the need to transfer vast datasets between sites. This type of decentralised approach is termed federated learning as the model is run locally at the edge, keeping sensitive data locally rather than transmitting it between systems. This increases security and privacy, as the local data remains private and is ultimately anonymised in the global model. The aim was to use AIDE clusters at existing Trusts alongside smaller federated learning clusters at new NHS Trusts, to create a shared data network of ten Trusts across London.
The AI Centre ultimately developed the Federated Learning Interoperability Platform (FLIP) to accelerate model training by using multiple datasets from numerous clusters at NHS Trusts. You can read more about this follow-on project in our FLIP case study.
The Scan Partnership
Scan has been the glue between the collaborative partners at every stage of this multi-year, consistently-evolving project. Coordination and communication with KCL, the AI Centre, NVIDIA, NetApp, Run:ai and the wider NHS Trust community has proved critical in the smooth delivery of each element of this GPU-accelerated solution, enabling improved diagnostics and patient care for ten million NHS users.
Scan initiated the guided POC and was instrumental in design of the first DGX cluster supporting its installation, upgrades and monitoring. For the AIDE clusters, Scan and its in-house 3XS Systems division developed a bespoke NVIDIA-certified EGX server to integrate with NVIDIA servers and networking, again installing and maintaining on each NHS site. All these hardware deployments have been further supported by Scan's system engineers and data science consultancy team.
Project Wins
Accelerate diagnosis from CT and MRI scans with real-time AI analysis
Enhanced detection and faster diagnosis of prostate cancers and stroke conditions
Image analysis in real-time for improved clinician-patient interaction at point of care
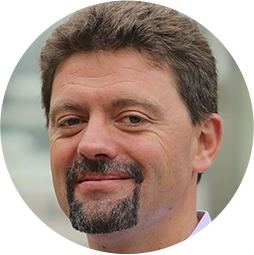
Prof. Sebastien Ourselin
Head of Biomedical Engineering & Imaging Sciences, KCL
"It has been fantastic working with these companies, especially through the guidance of Scan, who have been not only supporting the decisions of what solutions we should embed in our NHS Trust, but to put them together to build a turnkey solution."
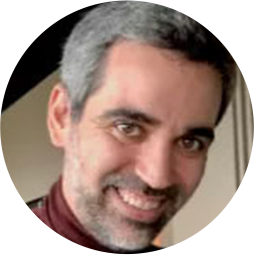
Dr M. Jorge Cardoso
CTO, London Medical Imaging and AI Centre for Value Based Healthcare
"With Run:AI we've seen great improvements in speed of experimentation and GPU hardware utilisation. Reducing time to results ensures we can ask and answer more critical questions about people's health and lives."
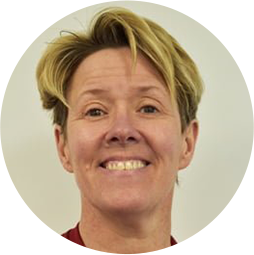
Beverley Bryant
CIO, Guy's and St Thomas' and King's College Hospital NHS Foundation Trusts
"We are delighted at the research and input from our consortium members. Working with a technology partner has expedited the launch of AIDE. This is just the beginning as we support the transformation of clinical diagnosis and treatments to be shared across the NHS and exported internationally"
Speak to an expert
You've seen how Scan continues to help Kings College London and the AI Centre for Value Based Healthcare accelerate medical diagnosis through AI. Contact our expert AI team to discuss your healthcare AI project requirements.
phone_iphone Phone: 01204 474210
mail Email: [email protected]