Oxford Robotics Institute
Using AI to Advance Robot LearningPublished 4 Dec 2023
From Primates to Robots:
Emerging Oscillatory Latent-Space Dynamics for Sensorimotor Control
Project Background
Understanding the intricacies of locomotion and manipulation is a key driver for both neuroscientists and roboticists - recent work in neuroscience has revealed that locomotion in primates is controlled by oscillations in monkey’s motor cortex [1]. These oscillations are observed in low-dimensional projections of neural populations in the motor cortex while the monkey is performing rhythmic movement such as swimming and walking and are also present with linear limb movement such as reaching. The utilisation of generative models in the field of robotics has revealed that robot locomotion can also be represented as oscillatory signals in low-dimensional representations. The ORI research team had previously created a structured latent-space for locomotion utilising a deep generative model in order to control a real quadruped robot [2,3]. The team wanted to explore the structure of the learnt latent-space for locomotion and show that key gait-specific properties emerge automatically.
Project Approach
The ORI team hypothesised that the oscillations which exist in latent space and in the motor cortex are a result of their structures. Since they were unable to artificially construct a motor cortex in the lab, they opted to create a learnt latent-space. To begin, a variational autoencoder (VAE) was trained for the quadruped and for the robotic arm manipulator, using a history of robot states including joint positions, torques and contact forces. Then the latent-space structure was investigated in two ways - firstly, trajectories from the test dataset were encoded and the resulting trajectories inspected; secondly, a short oscillatory trajectory was injected into latent space and decoded. The decoded trajectory was sent to a tracking controller and the robot’s movements were visualised. Finally, the team intended to artificially impair (lesion) the locomotion VAE in order to investigate how the latent-space trajectories map to the resulting robot trajectories.
Project Results
The ORI team found that the learnt latent-spaces were straightforward to inspect compared to the motor cortex. Therefore, they probed the latent-space structures to investigate the structure and compare the resulting trajectories to those observed in motor cortex. Additionally, they modulated the signals injected into the latent-space to discover how these affected the robot’s movement.
As seen in the diagram below, the locomotion trajectories in latent-space form a periodic cycle where the time period of the red signal in (c) controls the cadence of the robot and the amplitude governs the footstep height. The blue signal is inferred from the robot states and controls the footstep length of the robot, and is π/2 out of phase with the red signal.
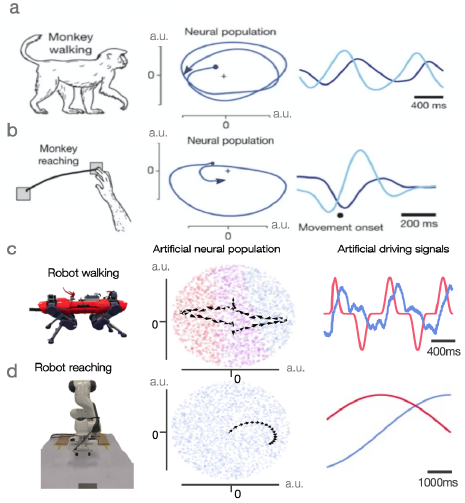
They repeated this experiment for manipulation, shown above in (d) and found that two sine wave oscillations, where the latter is also π/2 out of phase with the first, can solve reaching tasks. This resembled what was found in previous research [1], shown above in (b), where a cyclical pattern can be seen, constructed from two slightly out of phase periodic signals for both reaching and locomotion. At this stage the team could be sure whether this was a curious resemblance or something more fundamental.
The team then extended the analogy between the motor cortex and the latent-space by artificially impairing the locomotion decoder - something that cannot be done on a live motor cortex on both practical and ethical grounds, but was possible within the confines of their models. They utilised the same oscillatory drive signals and found that two pairs of legs are dragged along the ground and no longer take swing steps. The same sets of legs failed together since the locomotion is conditioned on the trot gait where the left front and right hind move together. They then encoded the resulting motion and noticed how the oscillations in latent-space changed shape and were no longer symmetrical, as shown in the diagram below.
Conclusions
There were visual similarities between the oscillations found in the primate’s motor cortex and the robot’s latent-spaces. Unlike the motor cortex, the ORI team was able to interrogate the structure of the latent space. In doing so, they found that emergent properties of locomotion and the reaching workspace become embedded in the space and as a result, they were able to generate new movements unseen during training. Furthermore, locomotion, cadence and footstep height were disentangled into separate orthogonal dimensions of the latent-space, facilitating the blending between dynamic trot gaits. This was further verified by the ability to lesion their VAE models to observe how the latent-space trajectories degraded.
Further information on these robotic experiments and the results can found by accessing the full research paper.
References:
[1] Churchland, M. M., Cunningham, J. P., Kaufman, M. T., Foster, J. D., Nuyujukian, P., Ryu, S. I., & Shenoy, K. V. (2012). Neural population dynamics during reaching. Nature, 487.
[2] Mitchell, A. L., Merkt, W., Geisert, M., Gangapurwala, S., Engelcke, M., Parker Jones, O., ... Posner, I. (2022). Next steps: Learning a disentangled gait representation for versatile quadruped locomotion. IEEE International Conference on Robotics and Automation (ICRA).
[3] Mitchell, A. L., Merkt, W. X., Geisert, M., Gangapurwala, S., Engelcke, M., Jones, O. P., . . . Posner, I. (2023). Vae-loco: Versatile quadruped locomotion by learning a disentangled gait representation. IEEE Transactions on Robotics, 1-16.
The Scan Partnership
The Scan AI team have been supporting ORI research projects as an industrial member for the past three years. Scan provide a hardware cluster of NVIDIA DGX servers, an NVIDIA EGX server and AI-optimised PEAK:AIO NVMe software-defined storage, to further robotic knowledge and accelerate development. This cluster is overlaid with Run:ai Atlas software in order to virtualise the GPU pool across the compute nodes to facilitate maximum utilisation and to provide a mechanism of scheduling and allocation of ORI workflows’ across the combined GPU resource. Access to this infrastructure is delivered via the Scan Cloud platform, hosted in a secure UK datacentre.
"We are delighted to have Scan as part of our set of partners and collaborators who are equally passionate about advancing the real-world impact of robotics research. Integral involvement of our technology and deployment partners will ensure that our work stays focused on real and substantive impact in domains of significant value to industry and the public domain.”
Ingmar Posner - Principle Investigator & Professor of Applied AI, Applied Artificial Intelligence Lab (A2I) at the Oxford Robotics Institute
Related content
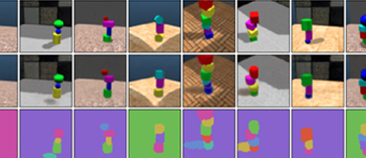
Using AI to Advance Robot Learning
Helping robots to be able to sense and interpret their surroundings in order to perform their intended tasks.
Read more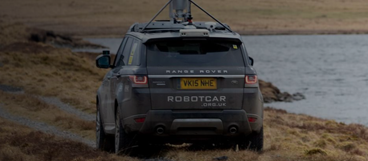
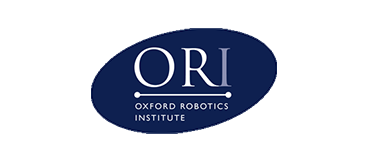