Our Aim
To provide you with an overview on New And existing technologies, hopefully helping you understand the changes in the technology. Together with the overviews we hope to bring topical issues to light from a series of independent reviewers saving you the time And hassle of fact finding over the web.
We will over time provide you with quality content which you can browse and subscribe to at your leisure.
TekSpek 's

NVIDIA DGX-1 Supercomputer
Date issued:
The increase in processing speed for computers has opened up new avenues of research and provided deeper insights into solutions for complex problems. Such power has been harnessed to accelerate research into medicine, better predict weather patterns, perform hugely complex calculations for the oil and gas industry, and a whole host more.
One area that has especially benefitted from a fantastic amount of horsepower is machine learning, where through a process of training and inference a machine is able to gain what humans consider to be artificial intelligence.
Computers take a multi-layer approach to training. For example, with respect to machine learning, or deep learning, for image recognition, the first layer may look for circles constituting a face and assign a weight to them, the next may look for particular edges, then the next layer will look for what amounts to a nose. Each layer passes on the learnings and weights to the next until the final, weighted layer calculates whether it's looking at a face or not. In other words, a computer does not see a face in the way humans do; it builds up layers of characteristics that enable it to determine whether all the attributes lead to a face.
Once there has been enough training data such that each output is deemed correct, algorithms look at a new image and then infer whether it's a face or not by calculating the probabilities. The same rules apply to any deep learning (or machine learning), so for speech recognition, disease spotting and so forth. Indeed, so good is machine learning in this respect that, once trained, it can match dermatologists in diagnosing skin cancer accurately. However, training huge datasets takes a huge amount of time and resources, but it just so happens that one part of the computing landscape, the GPU, is ideally suited to both heavy-duty training and fast inference.
The NVIDIA DGX-1 Deep Learning machine
GPU leader NVIDIA realised some time ago that GPUs were the best all-round candidates for deep learning that in turn has lead to the burgeoning artificial intelligence industry. Having potent hardware capable of sifting through terabytes of data and extracting meaningful information is one thing, but such GPU chops need to be married to efficient software, too. NVIDIA believes that it has both covered, and in an effort to speed-up the adoption of deep learning for data scientists in academia and businesses alike, it launched the DGX-1 deep learning computer, touted as the 'essential instrument of AI research'.
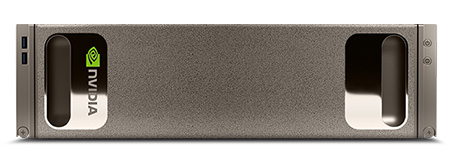
System specifications
GPUs | 8X Tesla V100 | 8X Tesla P100 |
---|---|---|
TFLOPS (GPU FP16) | 960 | 170 |
GPU Memory | 128 GB total system | |
CPU | Dual 20-Core Intel Xeon E5-2698 V4 2.2 GHz | |
NVIDIA CUDA® Cores | 40,960 | 28,672 |
NVIDIA Tensor Cores (on V100 based systems) | 5,120 | N/A |
Maximum Power Requirements | 3200 W | |
System Memory | 512 GB 2, 133 MHz DDR4 LRDIMM | |
Storage | 4X 1.92TB SSD RAID 0 | |
Network | Dual 10 GbE, 4 IB EDR | |
Software | Unbuntu Linux Host OS See Software Stack for Details |
|
System Weight | 134 lbs | |
System Dimensions | 866 D x 444 W x 131 H (mm) | |
Operating temp range | 10 - 35°C |
The fully-built DGX-1 'supercomputer-in-a-box' is outfitted with a choice of either eight Tesla P100 or eight Tesla V100 GPUs. In the case of the former, the Tesla P100 accommodates eight cards each equipped with 3,584 general-purpose Cuda cores and specialised NVLinks links between the GPUs to ensure that machine bandwidth is not stifled by older PCI Express. Such a machine offers 170 TFLOPS of single-precision performance, favourably comparing to an order of magnitude less from the best-performing CPUs of today.
Yet NVIDIA also realises that whilst the GPU is a prime candidate for deep learning, more can be done to speed calculations up further. The next generation of NVIDIA GPU architecture is called Volta and it harnesses what NVIDIA calls Tensor cores for staggering speed-ups. Paired alongside 5,120 cores inside each Volta-based Tesla V100 GPU, 640 Tensor cores combine to offer a machine-wide 960 TFLOPS of single-precision performance, or over 5x the amount of horsepower present in the Tesla P100 DGX-1 machines.
DGX-1 Architecture Advancements |
||
---|---|---|
System Level Specifications | NVIDIA DGX-1 with Tesla P100 | NVIDIA DGX-1 with Tesla V100 |
TFLOPS (FP16) | 170 | 960 |
CUDA Cores | 28,672 | 40,960 |
Tensor Cores | -- | 5,120 |
NVLink vs PCIe Speed-up | 5X | 10X |
Deep Learning Training Speed-up | 1X | 3X |
What's more, keeping all this data-crunching moving around the system, NVIDIA has designed a next-generation interconnect that enables up to eight Tesla V100 accelerators to be connected at speeds of up to 300GB/s, or 10x what's provided by the fastest PCIe today.
NVIDIA DGX-1 delivers 96X faster training
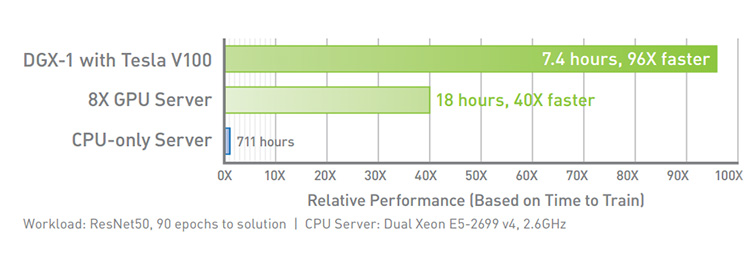
The sum of these changes means that a Tesla P100-equipped DGX-1 is about 40x faster than a CPU-only server at the computationally-expensive and long training element of deep learning. The Tesla V100 version, meanwhile is almost 2.5x faster than the base model, and it all works straight of the box.
Part of the reason for such easy deployment rests with NVIDIA spending considerable time and resource architecting the underlying software known as the DGX Software Stack. It plugs right into popular frameworks such as Caffe, Tensorflow, Torch and mxnet, and uses NVIDIA's Digits software as a base.
Deep learning is a fast-evolving part of high-performance computing. NVIDIA's combined expertise in hardware and software provides the ideal platform for data scientists to investigate the benefits of deep learning for a wide variety of applications. Scan Computers is proud that it has full NVIDIA NPN Accelerated Computing certification. Head on over to our 3XS site to learn more about Deep Learning to see how Scan Computers can facilitate deep learning for you.